Research Associate / PhD Student
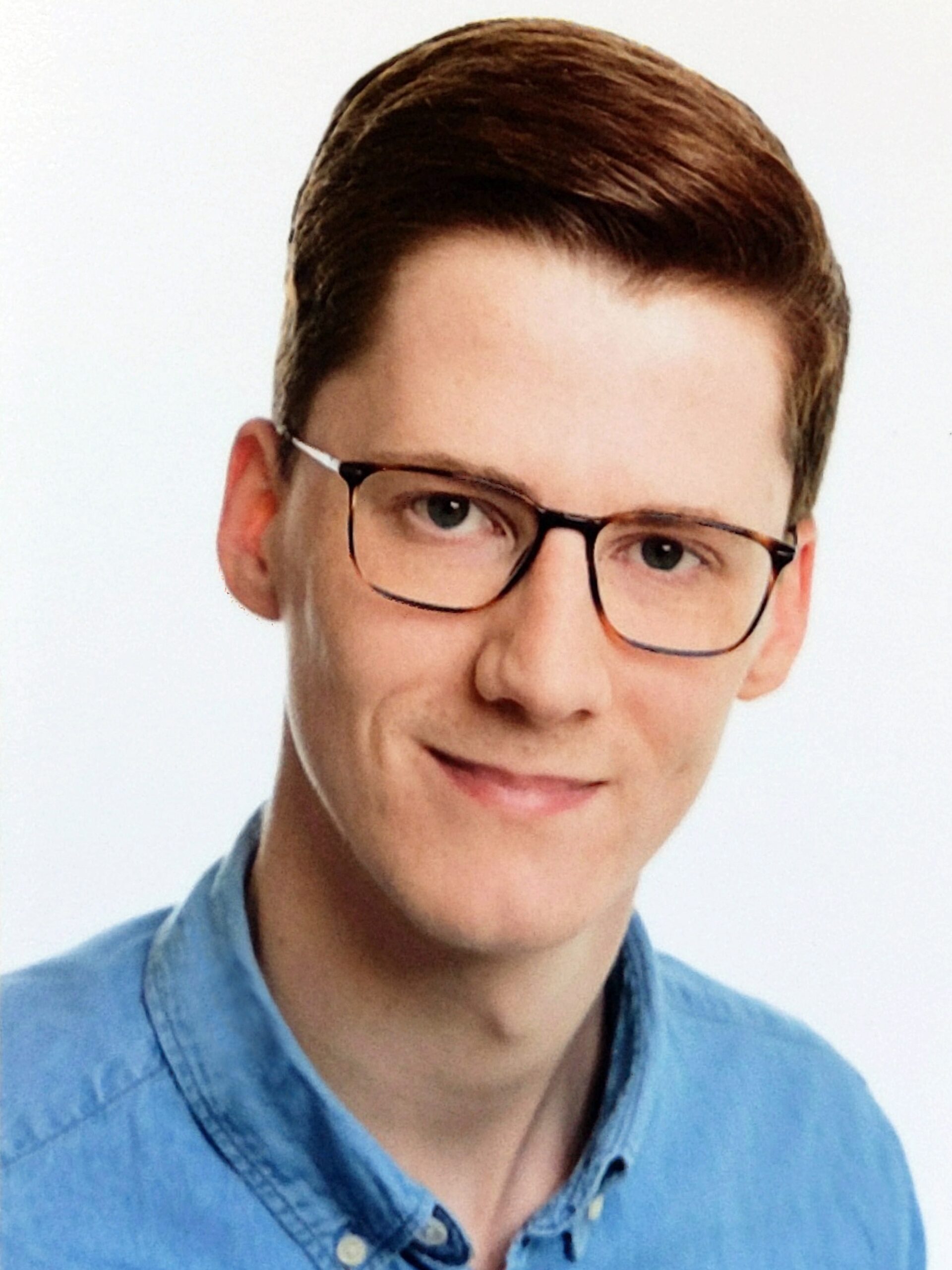
Nico Geisler, M. Sc.
Research Associate / PhD Student
Tel.: +49 (0) 9421 187-439
Mail: nico.geisler@tum.de
TUM Campus Straubing
Uferstraße 53
94315 Straubing
Uferstraße 53, Room: 02.3505.110
Vita
Employment History
- Since 09/2022
- PhD Student & Research Associate, Professorship for Bioprocess Engineering, Technical University of Munich, Campus Straubing for Biotechnology and Sustainability
- 06/2022 – 08/2022
- Research Assistant, Institute of Biochemical Engineering, Technical University of Braunschweig
- 01/2020 – 03/2021
- Student Assistant, Institute of Biochemical Engineering, Technical University of Braunschweig
- 01/2019 – 06/2019
- Student Assistant, Institute of Automotive Engineering, Technical University of Braunschweig
- 05/2018 – 07/2018
- Student Assistant, Institute of Genetics, Technical University of Braunschweig
Education
- 10/2019 – 05/2022
- Master of Science in Biotechnology, Technical University of Braunschweig
Thesis: “Sustainable production and structural analysis of bacterial cellulose and its application in fabric reinforced hybrid materials” - 09/2019 – 12/2019
- Master of Science in Biotechnology, Academic Exchange, University of Waterloo, Canada
- 10/2016 – 09/2019
- Bachelor of Science in Biotechnology, Technical University of Braunschweig
Thesis: “Metabolischer Crosstalk zwischen verschiedenen Clostridioides difficile Stämmen und einer humanen Darmkrebs-Zelllinie”
research project
The research focuses on having optimal control of industrial fermentation processes that are affected by batch-to-batch fluctuations when using hydrolysates derived from agricultural residues. Unlocking the full potential of bioprocess data, enabling faster troubleshooting, enhancing process automation and reducing the need for time-consuming and costly offline measurements by development of continuously trained, validated and improved hybrid model-based soft-sensors is the key objective. The process control involves the combination of real-time data from hardware sensors with specifically designed models to predict non-measurable parameters online.